Indexing
NumPy에서 indexing은 4+1 가지 방식을 따름.
- scalar를 이용한 indexing ( simple indexing ) :
array[0]
- slicing
- boolean mask :
array[array > 1]
- fancy indexing : vectorized indexing. index들을 element로 가지는 array를 넘겨줌.
- combined indexing : 앞서 4가지가 조합된 indexing
fancy indexing의 경우,
PyTorch 에서는 Tensor-based Indexing 또는 Advanced Indexing이라고도 불림.
scalar를 이용한 indexing과 slicing, boolean mask를 이용한 indexing은 다음 글을 참고:
[DL] Tensor: Indexing <Simple, Slicing, Fancy, Boolean Mask>
numpy나 pytorch, tensorflow의 텐서들도파이썬의 list 또는 tubple 에서의 indexing과 slicing이 거의 그대로 사용됨.2023.07.12 - [Python] - [Python] list (sequence type) : summary [Python] list (sequence type) : summarylist는 ordered
ds31x.tistory.com
Fancy Indexing
image(or matrix)등에서 높이 (row, height), 넓이 (column, width)에 대해,
- 접근하고자하는 pixel (or entry)들의 index를
- square bracket 안에 np.array 객체들 (=index array)로 넘겨주는 방식임.
주의할 점은
fancy indexing으로 넘겨지는 array의 shape에 따라
결과 array의 대응하는 axis의 shape가 결정됨
(앞부분의 shape가 index array의 shape와 같아짐.)
다음 자료의 fancy index 부분과 반드시 같이 볼 것
[DL] Tensor: Indexing <Simple, Slicing, Fancy, Boolean Mask>
NumPy나 PyTorch, Tensorflow의 텐서들도파이썬의 list 또는 tubple 에서의 indexing과 slicing이 거의 그대로 사용됨.2023.07.12 - [Python] - [Python] list (sequence type) : summary [Python] list (sequence type) : summarylist는 ordered
ds31x.tistory.com
간단하게 1차원에서 확인한다면 다음과 같음.
import numpy as np x = np.array([0,1,2,3,4,5,6,7,8])
Fancy indexing은 다음과 같이 index로 구성된 array를 index위치에 넘겨줌.
fidx = [3, 7, 1] x[fidx] # array([3, 7, 1])
Fancy indexing의 특징 중 하나는 넘겨준 index array의 shape에 맞추서 결과 ndarray가 만들어진다.
fidx =[[3, 7], [4, 2]] fidx = np.array(fidx) x[fidx] # array([[3,7],[4,2]])
fidx
가 2×2 matrix이므로x[fidx]
도 2×2 matrix가 됨.
Fancy indexing에서 각 axis에 대한 index를 따로 지정하는 경우도 매우 많음.
x = x.reshape((3,3)) ridx = np.array([0,1,2]) cidx = np.array([2,0,1]) x[ridx,cidx] # array([2, 3, 7])
강력한 점은 broadcasting rule을 통해 해당 index들의 array들이 최종 index들의 ndarray가 된다는 점임.
x[ ridx[:,np.newaxis], cidx]
결과는 다음과 같음.
array([[2, 0, 1], [5, 3, 4], [8, 6, 7]])
ridx
가 3×1 이므로cidx
와 broadcasting이 이루어져 최종 idx는 다음과 같음.
[(0,2)(0,0)(0,1)(1,2)(1,0)(1,1)(2,2)(2,0)(2,1)]
Broadcasting은 다음을 참고
2022.09.27 - [Programming/DIP] - [NumPy] Broadcasting
[NumPy] Broadcasting
ndarray와 scalar와 연산시킬때, 해당 ndarray와 같은 shape이면서 해당 scalar의 값을 가진 ndarray와 연산시키는 것처럼 자동으로 elementwise연산이 수행되는 기능. 다음과 같은 Rule에 따라 수행됨. Rules of..
dsaint31.tistory.com
각 축의 성분에 index tensor가 주어지지 않고 원본 tensor의 ndim보다 적은 수의 index tensor가 주어진 경우,
나머지 축들의 크기는 유지가 된다.
예를 들어
- 2×3×4의 원본 array a가 있는데 10×11 의 index array b가 주어진 경우,
- 3개의 축 중에서 1개의 축에만 index array가 주어졌으므로,
- a[b]의 shape는 10×11×3×4가 된다.
주의:
NumPy에서는 index array를 list로 해도 완전 동일하게 동작하지만,
PyTorch에서는 list를 index array로 사용하면 동작이 조금 다르다.
PyTorch의 tensor에서 사용할 경우 index array로 torch.tensor를 사용해야 앞서 설명한 같은 동작이 이루어짐.
https://gist.github.com/dsaint31x/a5c5b11fc3e1d75e5a0e4fa51ef03842
dl_fancyindexing_list.ipynb
dl_fancyindexing_list.ipynb. GitHub Gist: instantly share code, notes, and snippets.
gist.github.com
사실 PyTorch에서 nested list는 axis-0가 unpacking되어 들어가는 구조임. 위의 예제 ipynb 참고할 것.
Combined Indexing
Broadcasting을
반드시 이해하고 나서 읽어야 함.
fancy indexing은 나머지 indexing기법과도 조합하여 사용가능함.
# x는 3x2 matrix # [ [0,1,2], # [3,4,5], # [6,7,8] ] x[2, [2, 0, 1]] # combination between simple indexing and fancy indexing # 8,6,7
결과는 다음과 같음.
array([8, 6, 7])
# x는 3x3 matrix # [ [0,1,2], # [3,4,5], # [6,7,8] ] x[::-1, [0,2]] # combination b/w slicing and fancy indexing
결과는 다음과 같음.
array([[6, 8], [3, 5], [0, 2]])
boolean mask와도 조합이 가능함.
# x는 3x3 matrix # [ [0,1,2], # [3,4,5], # [6,7,8] ] mask = np.array([True, False, True]) cidx = np.array([2,0,1]) x[mask,cidx[:,np.newaxis]]
위의 코드는 다음과 같은 코드임.
x[[0,2],cidx[:,np.newaxis]]
- 주의할 건, boolean mask의 경우, broadcasting을 할 때
True
인 부분들만이 실제로 shape에 카운팅이 됨. - 위의 코드에서 boolean mask가 3개의 요소로 보이나 broadcasting 규칙을 적용할 때는 실제로는 2개의 요소만을 가진 ndarray로 간주됨.
- 때문에 broadcasting에서 각 축의 number of elements(요소의 수)가 맞지 않아 문제가 발생 할 수 있음. 주의필요.
결과는 다음을 참고
[[2 8] [0 6] [1 7]]
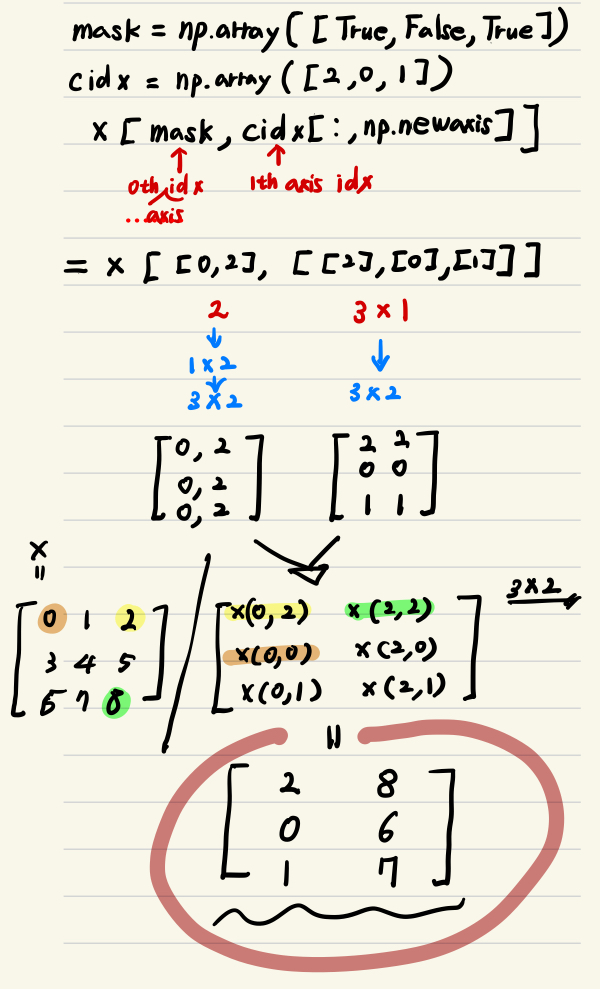
Fancy indexing을 이용하여 특정 item의 값을 변경할 수 있으나,
같은 index가 여러번 나온다고 해도 변경연산이 누적(혹은 반복)되진 않음.
x = np.arange(10) i = np.array([9, 1, 3, 9]) x[i] = x[i] +10 x
결과는 다음과 같음.
array([ 0, 11, 2, 13, 4, 5, 6, 7, 8, 19])
- 주의할 부분은 9를 index로 가지는 부분으로
- fancy indexing에서 2번 지정되었다고 10이 더해지는 연산이 2번 누적해서 일어나지 않음.
이같이 반복 처리를 하려면 at
메소드를 활용해야함.
x = np.arange(10) np.add.at(x,i,10) x
결과는 다음과 같음.
`array([ 0, 11, 2, 13, 4, 5, 6, 7, 8, 29])`
https://gist.github.com/dsaint31x/eb7a1fcc729ba3f349d7259002318976#file-fancyindexing-ipynb
FancyIndexing.ipynb
FancyIndexing.ipynb. GitHub Gist: instantly share code, notes, and snippets.
gist.github.com
[Summary] NumPy(Numerical Python)
파이썬 생태계에서 과학적 계산의 기본이 되는 라이브러리 NumPy는 파이썬에서 과학 계산과 수치 연산을 효율적으로 처리하기 위한 라이브러리 n-dimensional array(다차원 배열)인 ndarray 객체를 중
ds31x.tistory.com
'Programming > DIP' 카테고리의 다른 글
[DIP] Image Format (summary) (0) | 2022.12.05 |
---|---|
[DIP] Deconvolution: Richardson-Lucy deconvolution algorithm (0) | 2022.10.11 |
[DIP] OpenCV : Region of Interest (ROI) : Callback으로 구현. (0) | 2022.10.03 |
[DIP] opencv : Region of Interest (ROI) : cv2.selectROI (0) | 2022.10.03 |
[NumPy] Broadcasting (0) | 2022.09.27 |